In today's rapidly evolving business landscape, the integration of AI tools, particularly Large Language Models (LLMs), has become indispensable for enhancing operational efficiency and staying competitive. However, this technological advancement brings along significant responsibilities and challenges, necessitating a nuanced approach toward its adoption.
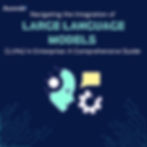
Businesses are increasingly leveraging LLMs to reshape customer interactions, refine sales strategies, and streamline administrative tasks. These AI-powered tools automate processes like lead scoring and provide instant call summaries with actionable insights, revolutionizing traditional workflows. Yet, as the potential of LLMs unfolds, it is imperative to acknowledge and address the accompanying risks.
The cornerstone of LLM (Large Language Models) integration lies in data – the most valuable asset in today's digital economy. As businesses entrust sensitive data to LLMs, they must navigate complex data custody issues. This demands a new playbook from solution providers, emphasizing the utmost importance of security and privacy.
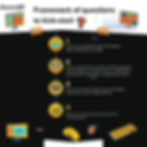
To navigate these challenges, initiating transparent conversations with technology partners is paramount. Here is a framework of questions to kick-start these discussions:
Data Privacy Assurance: How do you guarantee that LLM answers derive solely from my data?
Data Usage and Accessibility: Are my LLM interactions used for training purposes? How accessible is my data outside my organization, and does this comply with my data privacy policy?
Security Measures: How do you prevent confidential data exposure to unauthorized individuals, both outside and inside my organization, capable of manipulating LLMs?
Accuracy and Error Mitigation: How do you ensure LLM accuracy and address inaccuracies, such as hallucinations?
Performance Benchmarks: What are your performance benchmarks for LLM interaction?
Beyond these initial discussions, businesses must also consider broader ethical implications, regulatory compliance, and practical implementation strategies:
Ethical Considerations: Exploring the ethical implications of LLM usage, including biases and potential misuse, is essential for responsible AI deployment.
Regulatory Compliance: Adhering to regulatory frameworks such as GDPR (General Data Protection Regulation) or CCPA ensures that LLM integration aligns with legal requirements and protects consumer rights.
Practical Implementation: Providing actionable strategies for data anonymization, employee training, and cybersecurity measures to enhance the seamless integration of LLMs into existing workflows.
Moreover, incorporating real-life examples, case studies, and visual aids can enrich the discussion, making it more relatable and accessible to readers. At Ascendo, we have an AI workshop that caters to all of the above.
In conclusion, as Enterprises embark on the journey of integrating LLMs into their operations, prioritizing data privacy, security, and ethical considerations is paramount. Transparent communication with technology partners, adherence to regulatory guidelines, and a proactive approach toward addressing challenges will pave the way for successful and responsible AI adoption. Let us navigate this transformative era with caution, foresight, and a steadfast commitment to ethical AI practices.
Learn More